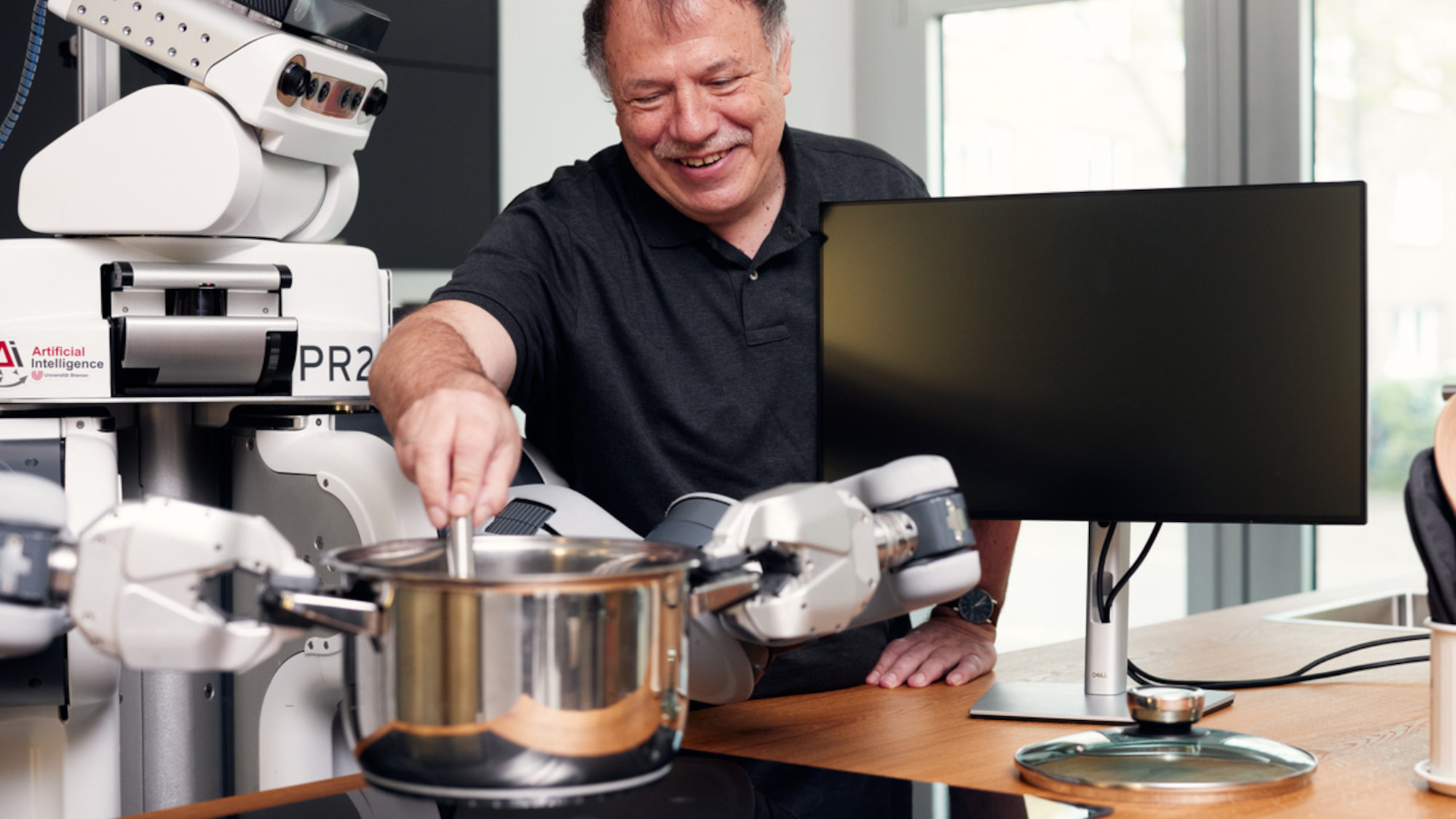
© Universität Bremen / Patrick Pollmeier
The Countless Variations of Pouring
How robots learn supposedly easy everyday tasks
Pouring tea water, pouring off pasta water, or filling a glass with cola – pouring liquids is a frequent task in everyday life. Therefore, robots have to master this if they are to help us in the household in the future. Although pouring is easy for us humans, it is very complex for robots. Michael Beetz, Professor of Artificial Intelligence in Faculty 3: Mathematics / Computer Science and Head of the Institute for Artificial Intelligence (IAI), is working on teaching them.
The reason Michael Beetz is so interested in pouring is not just due to its everyday relevance. “Pouring is also a challenging research topic because it is an underdetermined task,” he explains. This means that a request such as “pour cola into a glass” leaves a lot of room for interpretation and omits important information. What kind of glass should the robot choose, how viscous is cola, and how should pouring be adjusted when a liquid foams? The robot must know the answers to all these questions to avoid mistakes. Considering the many variations of pouring, Michael Beetz is faced with a major task. “My goal is for the robot to be able to pour any liquid out of any vessel, in any context,” he says.
2.5 Million Euros for Foundation Research
Michael Beetz’s work is in the field of foundation research. Since March 2023, he has received support for this from the European Research Council (ERC), which is funding his research with an Advanced Grant. 2.5 million euros are available to the computer scientist and his team over a period of five years. Researchers from the University of Bremen as well as from Bielefeld University and Paderborn University are involved in the research.
Michael Beetz and his team can only partially build on previous approaches and research findings in the field of artificial intelligence. “Systems like ChatGPT perform amazingly, but they lack a fundamental understanding of what they’re doing,” he explains. However, everyday robots should implement actions correctly right away, because mistakes could become dangerous – for example, if a robot spills hot water. He is therefore working on robots that consider different courses of action before selecting one, and that understand how and why they do something.
“It’s critical that robots understand the context of actions”
In order to achieve this goal, Michael Beetz wants to use the ERC funding to develop the “FAME” (Future-oriented cognitive Action Modelling Engine) software system. This software is designed to enable the robot to interpret videos, written instructions, and actions of people and to plan and implement its own actions based on this information. For example, when a robot observes a human pouring cola into a glass, it creates a virtual agent that resembles the person – a digital twin, so to speak. It reproduces and interprets the action with the digital agent, then transfers it to itself and saves the sequence for future tasks.
Before FAME can be implemented, the researchers in Michael Beetz’s team must work on various subtasks, such as the contextualization of information. “It is crucial that robots understand the context of actions and can automatically supplement missing information,” says Michael Beetz. “Imagine a robot watching a human pour off hot water from a pot of noodles. From its perspective, the robot may only be able to guess some movements or not recognize how much water is in the pot.” The information that the robot receives via its sensors is in this case not enough to capture the situation and process it for its own actions. Therefore, the robot should develop different scenarios and determine which of them is most likely.
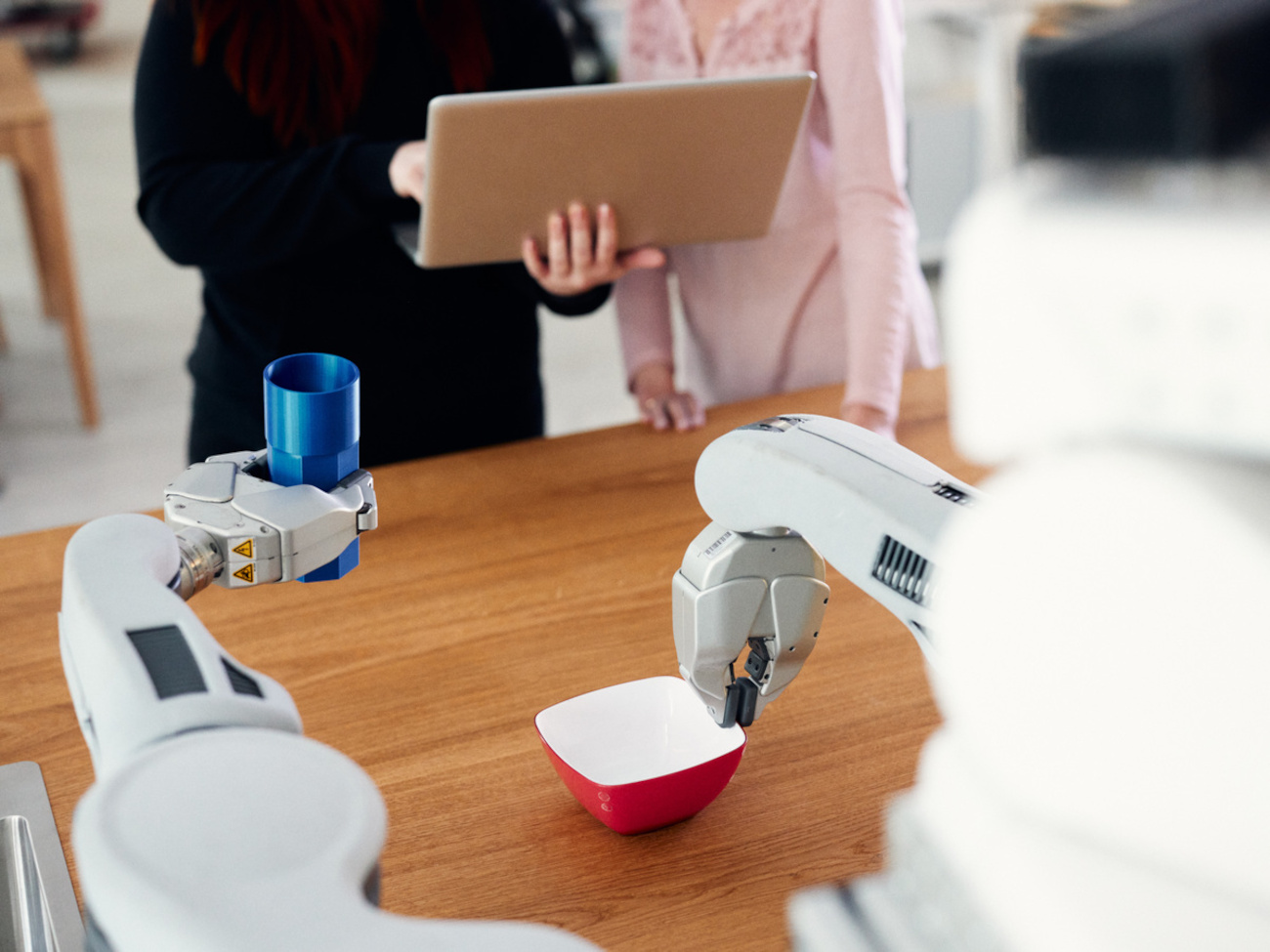
© Universität Bremen / Patrick Pollmeier
Robots must be capable of not just putting actions, but also instructions from people into context – as with the initially mentioned request to pour cola into a glass. To make this possible, the researchers in Michael Beetz’s team are working on another major topic: mental simulation. The robot creating a virtual model of a real observed situation is only the first step. Above all, it is important that the robot learns to relate the different individual actions to each other. “It must be able to establish causal relationships, for example between the tilt of the bottle and the flow rate of the liquid,” he outlines. “It can only use this information to plan its own actions later if it makes these connections.”
Achieving Goals with Interdisciplinary Cooperation
The Bremen team researchers are specifically investigating how robots process knowledge and perceive their environment and their actions. However, for the development of FAME, a better understanding of the interaction between robots and humans is crucial. The newly established Joint Research Center on Cooperative and Cognition-enabled AI (CoAI JRC) of the universities in Bremen, Bielefeld, and Paderborn assists with this part. In addition, together with Professor Philipp Cimiano from Bielefeld University, Michael Beetz is researching how existing instructions on the internet can be converted into knowledge bases that can be used by robots.
The work on FAME also allows the researchers to see how successfully the human brain processes information and plans actions. “It is only when trying to reproduce this in robots that you realize just how tremendous the brain’s performance in so many varied and uncertain physical and social situations is,” states Michael Beetz.